The Institute of Education Sciences, the U.S. Department of Education鈥檚 primary research arm, today launched a $7 million project to identify and quickly scale up effective practices to help students recover academically from pandemic disruptions.
The LEARN network, for Leveraging Evidence to Accelerate Recovery Nationwide, is one of three new research initiatives geared to pandemic recovery in schools, with others focused on supporting and helping staff implement promising practices. But IES Director Mark Schneider believes it will take a widescale overhaul of education research and data to accelerate progress for the students who have fallen furthest behind.
Schneider spoke to Education Week about what鈥檚 needed to help students recover academically from the pandemic. This interview has been edited for length and clarity.
We鈥檝e been trying to find effective ways to help struggling learners catch up for decades. What鈥檚 different about how you will be using networks like LEARN?
Mark Schneider: I鈥檓 concerned that the pace of traditional educational research is just too slow. My analogy is that when COVID came, we did Operation Warp Speed [to develop a pandemic vaccine]. The federal government invested across several vaccine producers: They preordered millions of doses from all of them and promised distribution鈥攁nd the reason was that they were covering their bets.
What if instead we said, hey, why don鈥檛 you do pharmaceutical research like we do education research? Let鈥檚 give, you know, a couple million dollars to Moderna and then three, four, five years later it didn鈥檛 work鈥攂ecause most of [the attempted vaccines] don鈥檛 work. Then we鈥檒l give money to Johnson & Johnson for a couple years; then if that doesn鈥檛 work, we鈥檒l give it to Pfizer, etcetera. We鈥檇 have died using serial long-term investments, and with the stakes so high in COVID, [vaccine research] was never going to be like that. But that鈥檚 pretty much the way it is in education research.
If we are facing a crisis of the size that we鈥檙e facing, we can鈥檛 run serial five-year contracts. We have to fail fast. Run experiments fast; replicate the few things that work in different geographies and in different demographic groups. Rinse and repeat. That鈥檚 the model we have to be pursuing.
What do you think needs to change in our approach to understanding struggling students?
Schneider: Since No Child Left Behind in 2002, by law and by practice, we鈥檝e focused on proficiency, because the goal under NCLB was to wipe out 鈥榖elow basic,鈥欌 [That鈥檚 the lowest possible score on the National Assessment of Educational Progress.] [The goal was] to turn everybody into a proficient reader, writer, science, math [student]. Obviously, that didn鈥檛 happen, but because we were focused on getting everybody past the proficiency mark, we paid less attention than we should have to what was going on below basic. But the trend of the below-basic [students鈥 achievement] falling is something that鈥檚 gotten worse.
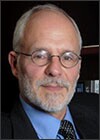
First of all, I think that NAEP has to change. Everything about NAEP is slow and cumbersome. And I don鈥檛 think there鈥檚 any disagreement in the NAEP world that paying more attention to below basic is essential. There鈥檙e not enough questions at the bottom of the distribution. And everybody knows that changing that is like redirecting a very big ship.
Many approaches to academic recovery rely on data use, and the pandemic caused a lot of disruption in state and federal data. How can we fix that?
Schneider: Look, we spent over $900 million building [], version one, right? And half of the money was spent in two years, 12 years ago, when these systems were built. I was commissioner of [the National Center for Education Statistics] in 2005 and signed the first two or three rounds of SLDS grants. So we鈥檙e talking ancient history. We need to think about a modern infrastructure for these incredibly important data systems and we need to think about how to integrate data across systems.
There鈥檙e worlds of information buried in data streams all over the place. We have to get much more sophisticated about using data. If we don鈥檛 figure out how more effectively to merge data and protect privacy, we鈥檙e leaving lots of chips on the table. At the same time, I鈥檝e been struggling with how to build a standard for ethical AI [artificial intelligence], because ... machine data can easily fall into all kinds of traps about building in prejudice and building in discrimination into our models.